Accurate search results and personalized recommendations are undisputedly the bedrock of modern ecommerce. As more businesses around the globe migrate online, the goal of every such company is simple — to help the user find what they’re looking for quickly and easily in order to enable maximum spending on the e-platform.
To make all of this possible — from search query relevance to ranking models and recommendation engines — machine learning (ML) is utilized. Three key aspects are required to see any ML system succeed: algorithms, hardware and the actual data. While the first two are readily available and pose no serious obstacles, data collection and labeling remain a major hurdle.
Data Labeling
How do we find and label data in a way that’s fast, accurate, affordable, and sustainable? Not only do businesses want to pay and wait less, but they also want reliable, high-quality data. And not on a one-off basis, but consistently — datasets and ML algorithms need to be updated and improved post-deployment.
A variety of options exist. One of the approaches is known as human-in-the-loop data labeling and falls under the general human-centric AI paradigm. This method makes use of human labelers and uses aggregation techniques, thereby producing large datasets resistant to the mistakes of individual performers.
Industry Trends and In-Demand Tasks
Among the many ecommerce tasks offered by human-in-the-loop third parties are:
- Recommender systems (RS)
- Search results relevance
- Online catalog improvement
- Price optimization
- Quality assurance and customer support
- Design, PR, and marketing
Let’s briefly look at three use cases based on this year’s trends:
Product search relevance.
This very common and important task is about making sure that when the user types a specific brand and/or model of a gadget in the e-platform’s search bar — be it a phone, tablet, or laptop — they actually get what they asked for, which sounds misleadingly simple.
Of course, the more accurate the result, the more satisfied the customer, and the higher the sales figures will be — and vice versa. With crowdsourced human-in-the-loop labeling, product search relevance can often climb up to over 90% and sometimes be as much as 60% faster than many in-house solutions. Crowd performers usually apply their human judgment in these tasks by rating search results from the most to the least relevant using a multiple choice questionnaire.
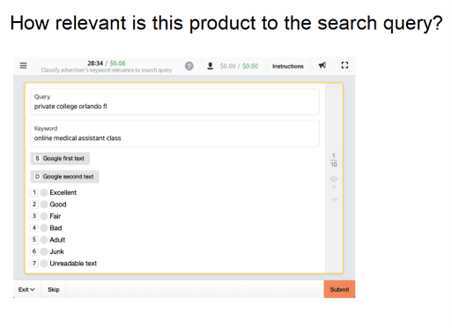
Recommendation of complementary items.
The challenge many e-platforms are constantly faced with has to do with the efficiency and accuracy of their recommender systems. This comes down to improving recommendation algorithms of complementary items and accessories in order to have the most relevant offers displayed to their customers. This is one of the most effective ways that ecommerce platforms get to improve their sales figures, grow and ultimately thrive.
Human-in-the-loop labeling has been shown to raise recommender system accuracy to around 90% and recall to around 75% in many cases. A typical data-labeling pipeline with an RS task may look something like this:
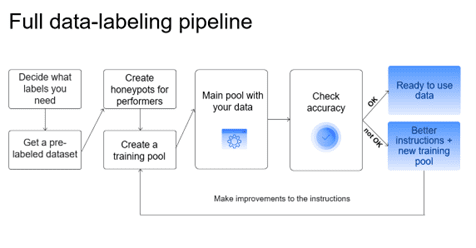
Human labelers are usually given photos of two products along with their technical specifications in the form of text. The contributors then need to determine whether the two products are compatible, i.e. whether one is an accessory or a possible complement to the other. A good example of this is a smartphone and a phone case, or any other combination of items that may normally belong together.
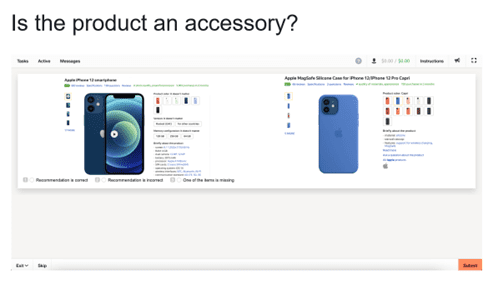
Serendipitous searches.
Another task that improves ecommerce sales in a different way is serendipitous searches, i.e. recommendation of new and unique goods for the customer’s incidental discovery. The goal here is not to let the user leave by offering them something exciting that they didn’t think they necessarily needed when they started shopping.
Serendipitous search accuracy with human-in-the-loop labeling can reach 92% accuracy in some situations. Thousands — sometimes tens of thousands — of items are human-labeled in such tasks, with the contributors being asked questions like “Is this item cool?,” “Do you find this product appealing?” and “Would you make a spur-of-the-moment purchase with this item?,” among others. Like most other ecommerce tasks, this can often be subjective, but that’s the whole point: only human labelers can weigh in on this type of user subjectivity in any meaningful way.
Main Takeaways
Human-in-the-loop data labeling is a solid candidate for a reliable and robust partner to the ecommerce and retail industry for the following reasons:
- The data ecommerce platforms require to improve their engines and algorithms needs to be both accurate and abundant. Human-in-the-loop labeling is fully capable of supplying both high-quality and high-quantity data to facilitate rapid and scalable AI development.
- Provided that both training algorithms and hardware solutions remain fixed variables available to most companies globally, it is precisely those companies that choose to focus on their data-labeling management that will win in the long run. Such companies are bound to do so by optimizing and minimizing their expenses, and ultimately expanding their business operations ahead of their competitors.
- The whole ecommerce and online retail industry is currently undergoing a major transformation — not only because it’s set to overtake offline retail in under two years, but also because more and more industry-specific tasks are becoming available for much less on many human-in-the-loop labeling platforms. This trend is expected to provide an additional boost to the ongoing growth of e-business.
Olga Megorskaya is the Founder and CEO at Toloka AI, a global data labeling solution. Previously, she developed data production infrastructure and implemented effective use of crowdsourced data labeling for ML-based products such as search, maps, voice assistants, self-driving cars and more. Megorskaya is a co-author of research papers on efficient crowdsourcing and quality control and has spoken at a number of top science conferences such as NeurIps, ICML, VLDB. In 2022, Megorskaya was featured in VentureBeat, Entrepreneur and Bloomberg as well as in the leading ML/AI publications.