Retail merchandising, like any field, has to keep up with a rapidly changing landscape. COVID-19 not only forced the shutdown of many brick-and-mortar retail companies but also changed purchasing habits for millions of customers.
These rapid shifts mean understanding consumer behavior and inventory levels like never before. Best practices and historical data about customer behavior cannot be used as an effective guide in the post-pandemic world. For any decision-maker adapting to change, making effective decisions on an ongoing basis is not easy.
A leading international beauty brand’s Director of Merchandise Planning with $10 billion in sales had to close most of her 1,000+ stores for months, with only her digital channels able to support sales. For the Director, this meant a difficult path in an uncertain time. These new trends meant a spike in online sales, but not enough resources to replenish stock in both stores and its digital fulfillment center (DC). She turned to Decision Intelligence (DI)for help.
Decision Intelligence in Retail Merchandising
This Director, like any decision-maker, evaluated data to project new trends, drive new promotions and create new strategies based on what she knows today to help her tackle the unknowns of tomorrow.
Advertisement
Unfortunately, there’s a gap between looking at raw data and forming good decisions. After all, many businesses have too much data as it is. With so much data being evaluated in static dashboards, it can be impossible to separate the signal from the noise to make optimal decisions.
Even in the new environment that COVID-19 brought about, this Director needed to take her data and apply her learnings to create real and actionable decisions in order to ultimately reach her business goals. Modeling off her previous data simply wouldn’t be good enough. This Director needed cognitive, prescriptive recommendations to guide her business.
The Decision Intelligence Difference
In the past, this Director would look over spreadsheets and ask herself what she needs to understand the business, or what promotions impact her margins. She had historical information to work off of and had to apply previous leanings to model out potential insights. Not only was this process manual and time-consuming, but it had inherent weaknesses. Among them was the fact that these ways of working left her ill-prepared to manage a sudden shift, like the Director experienced in the face of COVID-19. Not to mention that it was difficult to make sense of the output of various dashboards and data models.
With a strong DI system, the Director can simply ask a question like: “Show me week over week sales for Men’s Polo Shirts” and get a meaningful result.
Rather than just giving the Director more raw data, a proper intelligence system can give her pertinent insights into her business:
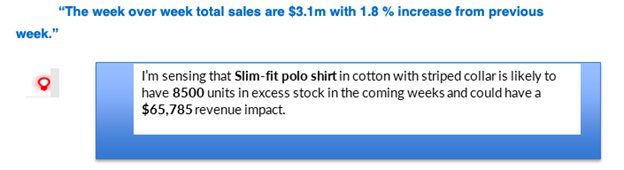
So: what just happened here?
A decision intelligence tool can apply a simple and conversational natural language interface to deliver an actionable recommendation — all without further prompting or input by the Director herself.
This flow encourages decision-makers to not only collect data but to actually parse and apply it in natural ways. For example, after receiving this insight a follow up question could be:
“What promotions should I run for the upcoming season?”
“I’m sensingaBOGO20 Promotion for this category with a potential recovery of $65,785.”

Rather than abstract data collection, this already-inundated Director can instead get recommendations that she immediately needs based on the data she already has.
In summary, DI allows retail decision-makers to have deeper conversations about building on current sales and promotions while exploring new opportunities — all at the right time and in the right business context.
The Future of Decision Intelligence in the Retail Space
DI takes data and goes beyond insights into actionable recommendations, empowering retailers to make informed and impactful decisions. As constructed, any retailer could feed the software as many data points as possible. Then the DI system will parse through the data and define the products with as many attributes as possible.
Since DI is flexible and product-neutral, the capacity for SKU recommendations is enormous. DI can enable complex insights for a variety of products. For example, as long as the retailer can identify the scent profiles that go into a perfume (and define the characteristics of each scent within the data itself), the director could get to the bottom of why certain fragrances are selling and why others are not.
DI is especially promising in retail because it has the potential to ensure that the inventory will be in the right place at the right time. With DI, companies spend fewer resources holding excess stock and reduce the risks of product shortages or the need to heavily mark down excess stock.
Krishna Kallakuri is CEO of diwo. Prior to founding diwo, Kallakuri was a founding member and CEO of DataFactZ, one of the fastest growing analytics companies in the Midwest. He brings more than 15 years of analytic technology and senior management experience, and is passionate about applying analytics to solve business problems.